Build and Deploy Supervised, Unsupervised, Deep, and Reinforcement Learning Models: A Comprehensive Guide

In the rapidly evolving field of artificial intelligence (AI),machine learning models have emerged as powerful tools for solving complex problems and driving innovation. Among the various types of machine learning algorithms, supervised, unsupervised, deep, and reinforcement learning have gained significant prominence. This comprehensive guide provides a comprehensive to these techniques, empowering you to build and deploy models that can tackle challenging tasks and transform industries.
Supervised learning is a type of machine learning where a model is trained on a dataset of labeled data. The model learns to map input data to the corresponding output labels. Examples of supervised learning tasks include image classification, object detection, and regression.
- Labeled Data: Supervised learning requires a dataset where each data point is associated with a known label.
- Model Parameters: The model is characterized by a set of parameters that are adjusted during training.
- Loss Function: The loss function measures the difference between the model's predictions and the true labels.
- Optimization Algorithm: An optimization algorithm is used to update the model parameters and minimize the loss function.
Supervised learning has a wide range of applications, including:
5 out of 5
Language | : | English |
File size | : | 6766 KB |
Text-to-Speech | : | Enabled |
Screen Reader | : | Supported |
Print length | : | 252 pages |
- Image recognition and classification
- Object detection and segmentation
- Predictive analytics and forecasting
- Natural language processing
Unsupervised learning is a type of machine learning where a model is trained on a dataset of unlabeled data. The model learns to identify patterns and structures in the data without relying on explicit supervision. Examples of unsupervised learning tasks include clustering, dimensionality reduction, and anomaly detection.
- Unlabeled Data: Unsupervised learning does not require a dataset with labeled data.
- Data Representations: The model learns to represent the data in a meaningful way, often by reducing the dimensionality of the data.
- Clustering Algorithms: Clustering algorithms group similar data points into clusters.
- Dimensionality Reduction Techniques: Dimensionality reduction techniques reduce the number of features in the data while preserving important information.
Unsupervised learning has various applications, such as:
- Market segmentation and customer profiling
- Anomaly detection and fraud prevention
- Document and text clustering
- Image and video analysis
Deep learning is a type of machine learning that uses artificial neural networks (ANNs) to learn complex relationships in data. ANNs are hierarchical structures composed of multiple layers of connected nodes, allowing them to extract high-level features from data. Examples of deep learning tasks include image recognition, natural language processing, and speech recognition.
- Neural Networks: Deep learning models are based on neural networks, which mimic the structure and function of the human brain.
- Layers: Neural networks consist of layers, each performing a specific transformation on the data.
- Forward and Backward Propagation: During training, data is propagated through the network (forward pass),and errors are propagated back (backward pass) to adjust the model's parameters.
- Activation Functions: Activation functions introduce non-linearity into the network, allowing it to learn complex relationships.
Deep learning has revolutionized many fields, including:
- Computer vision and image processing
- Natural language processing and machine translation
- Speech recognition and analysis
- Healthcare and medical diagnosis
Reinforcement learning is a type of machine learning where an agent learns to navigate an environment by trial and error. The agent receives rewards or penalties for its actions and adjusts its behavior over time to maximize the cumulative reward. Examples of reinforcement learning tasks include game playing, robot control, and resource allocation.
- Environment: The agent interacts with an environment that provides rewards or penalties based on the agent's actions.
- Agent: The agent makes decisions and takes actions based on its learned policy.
- Reward Function: The reward function defines the agent's goal and provides feedback on its actions.
- Value Function: The value function estimates the expected future reward for a given state.
Reinforcement learning has promising applications in various domains, such as:
- Game playing and AI algorithms
- Robotics and autonomous systems
- Dynamic resource allocation and optimization
- Financial trading and portfolio management
Building and deploying machine learning models involves several key steps:
- Data Collection and Preprocessing: Collect and preprocess the data to ensure it is suitable for training the model.
- Model Selection and Training: Choose the appropriate machine learning algorithm and train the model on the prepared data.
- Model Evaluation: Evaluate the model's performance on a validation dataset to assess its accuracy and robustness.
- Model Deployment: Deploy the trained model to a production environment where it can make predictions or perform tasks.
- Model Monitoring and Maintenance: Regularly monitor the deployed model and make adjustments as needed to ensure optimal performance and address any changes in the data or environment.
Despite the significant potential of machine learning models, there are several challenges to consider:
- Data Quality and Availability: Poor-quality data or insufficient data can hinder the model's ability to learn and make accurate predictions.
- Model Complexity and Interpretability: Building complex models may lead to overfitting or difficulty in interpreting the model's decision-making process.
- Computational Resources: Training and deploying deep learning models can require significant computational resources.
- Bias and Fairness: Machine learning models can inherit biases from the training data, leading to unfair or discriminatory outcomes.
- Security and Privacy: Deploying machine learning models in production environments raises concerns about security and privacy of data.
To overcome the challenges of building and deploying machine learning models effectively, consider the following best practices:
- Ensure the quality and diversity of the training data.
- Start with simple models and gradually increase complexity as needed.
- Use techniques like cross-validation and regularization to prevent overfitting.
- Implement interpretability methods to understand the model's behavior and decision-making.
- Leverage cloud computing platforms for efficient training and deployment.
- Address bias and fairness by using appropriate data sampling and mitigation techniques.
- Implement robust security measures and data privacy protocols.
Mastering deep and reinforcement learning techniques empowers you to build and deploy powerful machine learning models that can address complex challenges and drive innovation across industries. This comprehensive guide provides a foundation for understanding these techniques, highlighting their key concepts, applications, and best practices. By embracing the principles outlined in this guide, you can confidently navigate the world of machine learning and harness its transformative potential for your organization.
5 out of 5
Language | : | English |
File size | : | 6766 KB |
Text-to-Speech | : | Enabled |
Screen Reader | : | Supported |
Print length | : | 252 pages |
Do you want to contribute by writing guest posts on this blog?
Please contact us and send us a resume of previous articles that you have written.
Book
Novel
Page
Chapter
Text
Story
Genre
Reader
Library
Paperback
E-book
Magazine
Newspaper
Paragraph
Sentence
Bookmark
Shelf
Glossary
Bibliography
Foreword
Preface
Synopsis
Annotation
Footnote
Manuscript
Scroll
Codex
Tome
Bestseller
Classics
Library card
Narrative
Biography
Autobiography
Memoir
Reference
Encyclopedia
Exodus
Odelia Chan
Michael E Mcgrath
Avijit Ghosh
Antonio Galbis
Royston Wood
Marc Humphrey
Stephen Dunn
Jhumpa Lahiri
Rebecca Barrow
A Hyatt Verrill
Ariel Kaplan
Neil Mcnerney
Nicola Carroll
Sharon Gosling
A Antonio
A Breeze Harper
Jeff Dwyer
Robert V Allegrini
Earl Browning
Light bulbAdvertise smarter! Our strategic ad space ensures maximum exposure. Reserve your spot today!
- Grant HayesFollow ·14.2k
- Jessie CoxFollow ·3.6k
- Banana YoshimotoFollow ·8.6k
- Jon ReedFollow ·4.9k
- Christian BarnesFollow ·4.9k
- David Foster WallaceFollow ·4.8k
- Jacob HayesFollow ·2.9k
- Alvin BellFollow ·12.5k
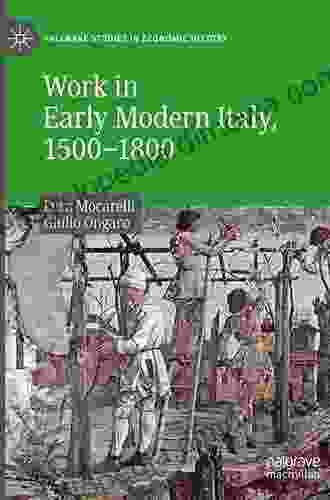

Work in Early Modern Italy 1500-1800: A Captivating...
: Unraveling the Enigmatic...


Iceland's Most Unusual Museums: A Quirky Guide to the...
Iceland is a land of natural wonders, from...
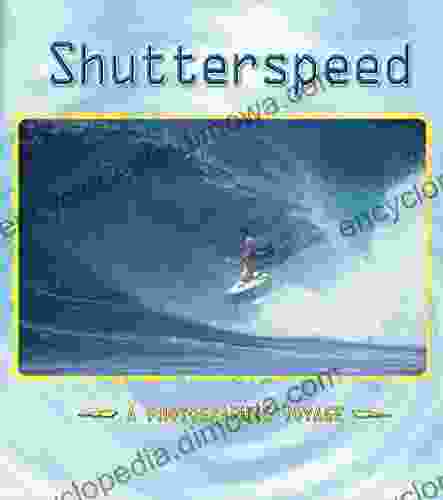

ShutterSpeed - A Photographic Voyage
Embark on an Unforgettable Photographic...
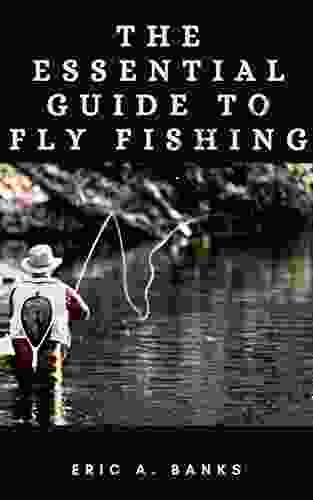

Unveiling the Secrets: Detailed Streamside Field Guide to...
Embark on an unforgettable fly fishing...


Hannah Montana: Reality Check Junior Novel 19
The Ultimate Behind-the-Scenes Adventure for...


Create More Than 30 Projects from Vintage Pieces: Unleash...
Embrace the Art of...
5 out of 5
Language | : | English |
File size | : | 6766 KB |
Text-to-Speech | : | Enabled |
Screen Reader | : | Supported |
Print length | : | 252 pages |